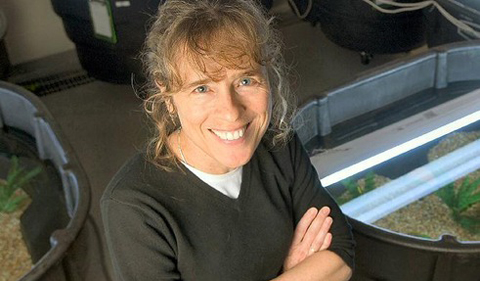
Dr. Molly Morris
The same technology used by forensic investigators to capture fingerprints also can help doctors predict whether patients might be at risk for developing diabetes, a new study published in the Journal of Diabetes Science and Technology.
A team of scientists and clinicians led by Dr. Molly Morris, Professor of Biological Sciences at Ohio University, has found that a technology called wavelet analysis can take detailed snapshots that reveal the level of asymmetry in an individual’s fingerprints. The study found that fingerprint asymmetry is a marker of type 2 diabetes in adults. In addition, the team discovered a connection between the irregular fingerprint patterns and patients with type 1 diabetes.
The use of fingerprints to diagnose diseases is currently a very active field of medical research, but this study—A New Method to Assess Asymmetry in Fingerprints Could Be Used as an Early Indicator of Type 2 Diabetes Mellitus— is the first to efficiently measure fluctuating asymmetry in fingerprints (differences in fingerprints between the corresponding fingers on the right and left hands) and show that it can be used to predict a person’s risk to develop diabetes.
The new study compared a ridge-counting technique with wavelet analysis and found that the latter approach provided a more accurate, detailed assessment of fingerprint asymmetry.
“There were individuals we couldn’t assess (through ridge counting) because of the type of fingerprints they had,” said Morris, a professor of biological sciences. “Wavelet analysis is almost like taking a picture of your fingerprint. It’s a more sophisticated analysis.”
To gather data for the study, Morris and Jay Shubrook, at the time a faculty member in the Ohio University Heritage College of Osteopathic Medicine and now with Touro University California, recruited 340 adult patients. Of those, 200 had been diagnosed with type 2 diabetes and 57 other subjects had type 1 diabetes. Fingerprints were taken and then analyzed by counting the number of ridges on the fingers and by using wavelet analysis.
More Accurate Than Genetic Testing
Bjoern Ludwar, an electrophysiologist with Longwood University and a former Ohio University postdoctoral fellow, had suggested the wavelet analysis method, which can represent the fingerprint image as a short string of numbers. This process can help researchers assess the asymmetry of the patient’s fingerprints more accurately than other methods, even when the fingerprints are rotated at different positions, he explained.
“The wavelet method takes a very global approach to comparing how similar two fingerprints are,” he said. “On the downside, it is technically a lot more complicated and it takes more computing power than simply counting ridges on fingers of the left and right hand.”
Current methods of diagnosing diabetes rely on physical signs of disease progression or expensive genetic testing.
“We really need to become better at addressing diabetes mellitus upstream—the earlier we can find people at risk, the more we can do to take action to help prevent these diseases,” Shubrook said.
Because an individual’s fingerprints are set at birth, they can provide a very early indicator for the propensity to develop diabetes, the team noted. Fingerprints are influenced by both genes and the environment, which could explain why the method was more accurate than genetic testing, the researchers added.
Ludwar plans to optimize the method to make the process available for commercial use, as the team hopes to create a mobile app that could identify at-risk individuals before they develop symptoms.
In addition to focusing on enhancements to the technology, in the next phase of the research the team will gather fingerprint data from a second, more ethnically diverse pool of patients in California, Morris said. The team hopes to recruit about 300 individuals, half of which will be patients over the age 40 without type 2 diabetes to broaden the control groups tested, she explained.
The team has filed for a provisional patent on the method.
The study was funded by Ohio University. Co-authors also include Evan Swingle of Ohio University and Mahelet Mamo of Longwood University.
Comments